Natural Language Processing (NLP) is a subfield of Artificial Intelligence (AI) that focuses on enabling machines to understand and process human language.
NLP involves developing algorithms and models that can analyze, interpret, and generate human language, including both written and spoken forms. In this article, we will discuss the role of NLP in AI, its history, techniques used, applications, challenges, and future prospects.
What is the Role of NLP in AI
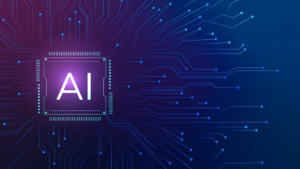
The role of NLP (Natural Language Processing) in AI (Artificial Intelligence) is to enable machines to understand and process human language. This is achieved through the use of algorithms and statistical models that allow machines to recognize patterns in human language and make predictions based on that data.
NLP is a critical component of AI as it enables machines to interact with humans in a more natural and intuitive way. It has numerous applications in various industries, including healthcare, finance, and customer service. As advancements in deep learning continue to improve the accuracy and efficiency of NLP models, its potential impact on the field of AI (Artificial Intelligence)is expected to grow even further.
Related: How Ai is Changing the Healthcare Industry
History of NLP (Natural Language Processing)
The history of NLP can be traced back to the early days of computing when scientists and researchers started exploring ways to make machines understand and generate human language.
The first significant milestone in NLP was the development of the first language translation program in 1954. Since then, several key advancements have been made, such as the creation of the first speech recognition system in the 1960s, and the development of the first chatbot, ELIZA, in the 1966s. In recent years, Natural Language Processing has made tremendous progress due to advancements in machine learning and deep learning.
Techniques used in NLP
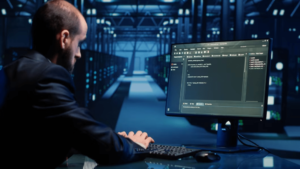
There are several techniques used in NLP that enable machines to process human language. Tokenization involves breaking down a sentence into its individual words or phrases.
Part of speech tagging is the process of identifying the grammatical components of each word in a sentence. Named entity recognition involves identifying and categorizing named entities such as people, organizations, and locations. Sentiment analysis is the process of identifying and categorizing the emotional tone of a piece of text. Dependency parsing involves identifying the relationships between words in a sentence.
Applications of NLP
NLP has numerous applications, ranging from text classification to machine translation, chatbots, and virtual assistants. Text classification involves categorizing text documents into predefined categories based on their content. Machine translation is the process of translating text from one language to another.
Chatbots and virtual assistants use Natural Language Processing to understand and respond to user queries. Sentiment analysis is used to analyze customer feedback and social media posts to gain insights into customer sentiment. Voice assistants use NLP to understand and respond to spoken commands.
Related: How ai can improve our lives in the Future
Challenges in NLP
Despite the progress made in Natural Language Processing, there are still several challenges that researchers face. Ambiguity in the language is one of the major challenges, as words and phrases can have multiple meanings depending on the context in which they are used.
Variation in language use across different regions and cultures is another challenge. Multilingualism is also a challenge, as machines must be able to understand and process multiple languages. Finally, lack of context in text data is a challenge, as machines need to be able to understand the context in which text is used to accurately process it.
Future of NLP
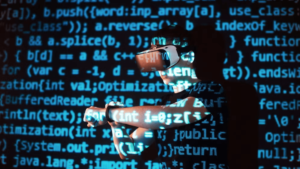
The future of Natural Language Processing (NLP) is promising, as advancements in deep learning are expected to revolutionize the field. Deep learning models, such as neural networks and transformers, are
expected to improve the accuracy and efficiency of NLP models. Additionally, the integration of NLP with other AI (Artificial Intelligence) technologies such as computer vision and robotics is expected to further expand its applications.
Natural Language Processing (NLP) is also expected to have a significant impact on various industries, including healthcare, finance, and customer service. In healthcare, NLP can be used to analyze medical records and identify patterns that can aid in diagnosis and treatment. In finance, NLP can be used to analyze financial reports and predict market trends. In customer service, NLP can be used to improve the efficiency and accuracy of chatbots and virtual assistants.
You May Like: How Mobile is Transforming the healthcare industry
In conclusion, NLP is a critical component of AI that enables machines to process human language. Its applications are numerous, and its impact on various industries is expected to be significant. Despite the challenges faced by researchers, advancements in deep learning and integration with other AI (Artificial Intelligence) technologies are expected to lead to further progress in the field.
FAQs
What is the difference between NLP and machine learning?
NLP is a subfield of machine learning that focuses specifically on enabling machines to process human language.
Can NLP be used to translate between all languages?
While NLP can be used to translate between multiple languages, there are still some languages that present unique challenges due to their complexity and variability.
How accurate are Natural Language Processing models?
The accuracy of NLP models depends on various factors, such as the quality of the training data and the complexity of the language being processed. However, with advancements in deep learning, the accuracy of NLP models is improving.
What are some of the ethical concerns surrounding NLP?
Some ethical concerns surrounding NLP include privacy issues related to the processing of personal data, bias in Natural Language Processing models, and the potential for misuse of NLP technology.
If you like this article please share it with others. Thanks